The Ultimate Guide to Labeling Tool Machine Learning
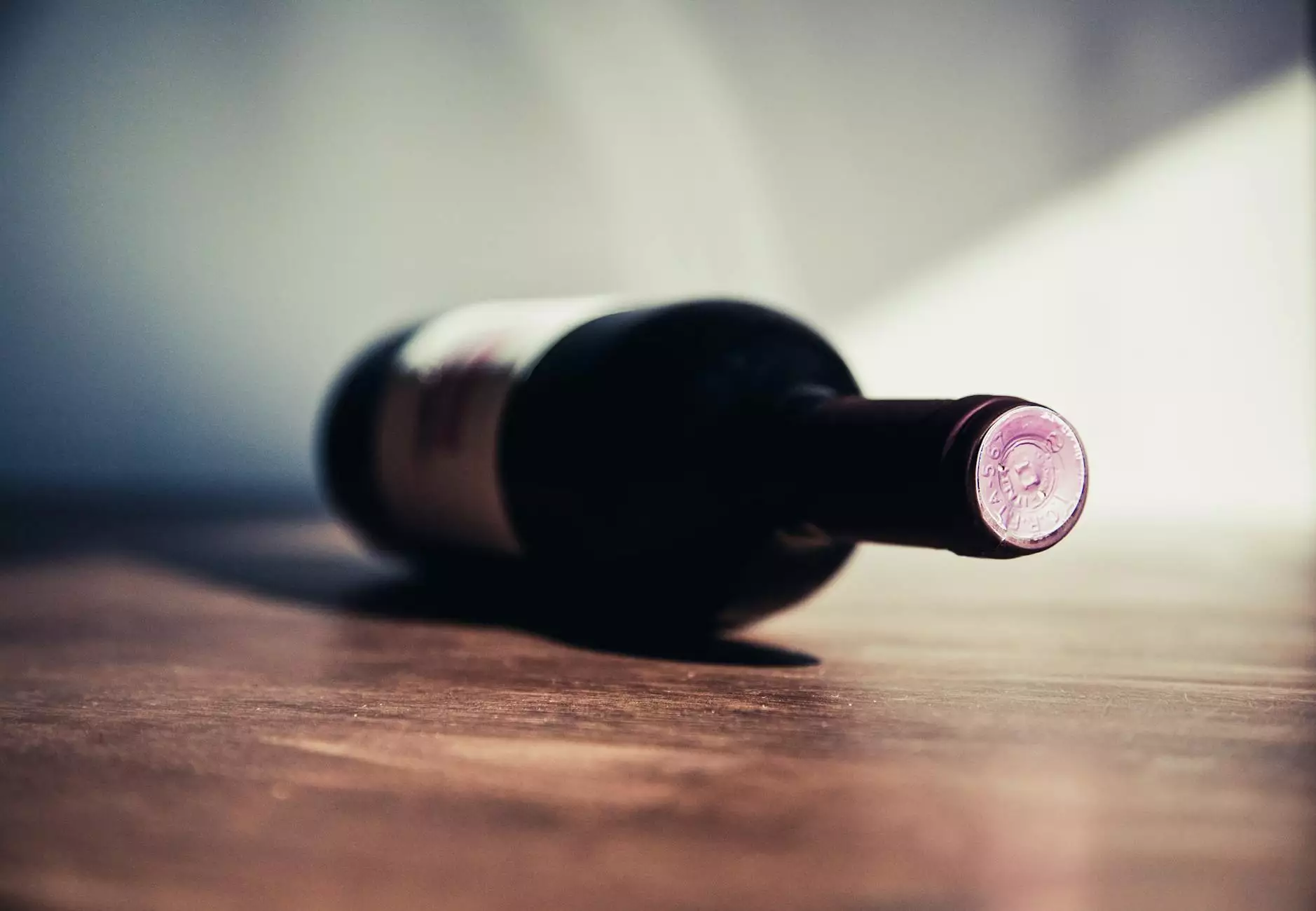
Understanding Labeling Tool Machine Learning
In today's digital landscape, labeling tool machine learning plays an essential role in transforming raw data into actionable insights. At its core, machine learning is about enabling computers to learn from and make predictions based on data. Labeling tools are instrumental in this process, as they help convert unstructured data into structured forms, thereby facilitating machine learning algorithms.
Importance of Data Annotation
Data annotation is a crucial step in the machine learning pipeline. By labeling data accurately, businesses can train models that perform specific tasks such as image recognition, natural language processing, and more. Without proper data annotation, these machine learning models may produce inconsistent or inaccurate results. Here’s why data annotation matters:
- Quality Insights: Well-annotated data leads to better performance of machine learning models, resulting in valuable insights.
- Enhanced Predictions: The accuracy of predictions significantly improves when models are trained on high-quality, annotated datasets.
- Time Efficiency: Automated labeling tools can streamline the data annotation process, saving time and reducing human error.
The Role of Labeling Tools in Machine Learning
Labeling tools are software applications that assist in the annotation of datasets. They streamline the process, enabling teams to focus on analysis rather than manual data entry. Here are some key functionalities of these tools:
- Automatic Tagging: Many labeling tools implement machine learning algorithms that assist in automatically tagging data, reducing manual workload.
- Collaboration Features: Modern tools allow multiple users to collaborate seamlessly, ensuring that data is annotated quickly and accurately.
- Support for Various Data Types: From images and videos to text and audio, advanced labeling tools can handle diverse data types effectively.
Key Features of Advanced Labeling Tool Machine Learning Solutions
When evaluating labeling tools, consider the following features that can significantly enhance your data annotation efforts:
- Intuitive User Interface: A user-friendly interface ensures that team members can quickly learn and utilize the tool.
- Integration Capabilities: Compatibility with existing workflows and systems is critical for smooth operations.
- Advanced Algorithms: Tools that incorporate the latest in machine learning algorithms can improve automation and accuracy.
- Quality Control Mechanisms: Features like validation checks and audit trails can help maintain data integrity throughout the annotation process.
Case Studies: Success Stories Using Labeling Tool Machine Learning
Numerous organizations have successfully leveraged labeling tool machine learning to optimize their data processes. Here are a few notable examples:
1. Retail Industry
A leading retail company utilized machine learning labeling tools to analyze customer feedback across multiple channels. By effectively annotating textual data, they were able to identify customer sentiment and enhance their product offerings based on customer preferences.
2. Healthcare Sector
In healthcare, a hospital network implemented annotation tools to label patient data for predictive modeling. By deriving insights from accurately annotated medical records, they improved patient outcomes through tailored treatments and early diagnosis strategies.
3. Autonomous Vehicles
The automotive industry has seen significant advancements in self-driving technology due to effective data annotation. By utilizing high-end labeling tools for image and video data from vehicle sensors, manufacturers have been able to train robust models that ensure safer navigation and improved decision-making for autonomous systems.
Challenges in Data Annotation and How to Overcome Them
Despite the advantages, businesses often face several challenges during the data annotation process. Here are common difficulties and solutions:
Inconsistency in Labeling
Inconsistencies can arise from different annotators interpreting data differently. To mitigate this:
- Clear Guidelines: Provide explicit labeling guidelines to ensure that everyone understands what is expected.
- Regular Training: Conduct training sessions to align annotators on standards and best practices.
Handling Large Datasets
Large datasets can become overwhelming. To streamline the annotation:
- Utilize Automation: Employ labeling tools that feature machine learning for automatic data tagging.
- Batch Processing: Process data in batches to make the workload more manageable for teams.
Choosing the Right Labeling Tool Machine Learning Provider
Selecting an appropriate labeling tool provider can be a game changer for your business. Here are factors to consider:
- Reputation and Experience: Look for providers with a proven track record in the industry.
- Client Testimonials: Evaluate feedback from existing clients to gauge user satisfaction.
- Scalability: Ensure that the tool can scale with your business growth.
Conclusion: The Future of Labeling Tool Machine Learning
As machine learning continues to evolve, the impact of labeling tool machine learning on data annotation practices will become even more significant. Businesses that embrace these tools will not only gain competitive advantages but will also unlock new opportunities for innovation and growth. By investing in robust labeling solutions like those from KeyLabs.ai, organizations can ensure their data operations are efficient, accurate, and future-ready.
Get Started Today!
To revolutionize your data annotation processes, explore the comprehensive features of labeling tools at KeyLabs.ai. Embrace the power of machine learning to unlock your data's full potential!