Enhancing Data Annotation with Bounding Boxes: A Comprehensive Guide
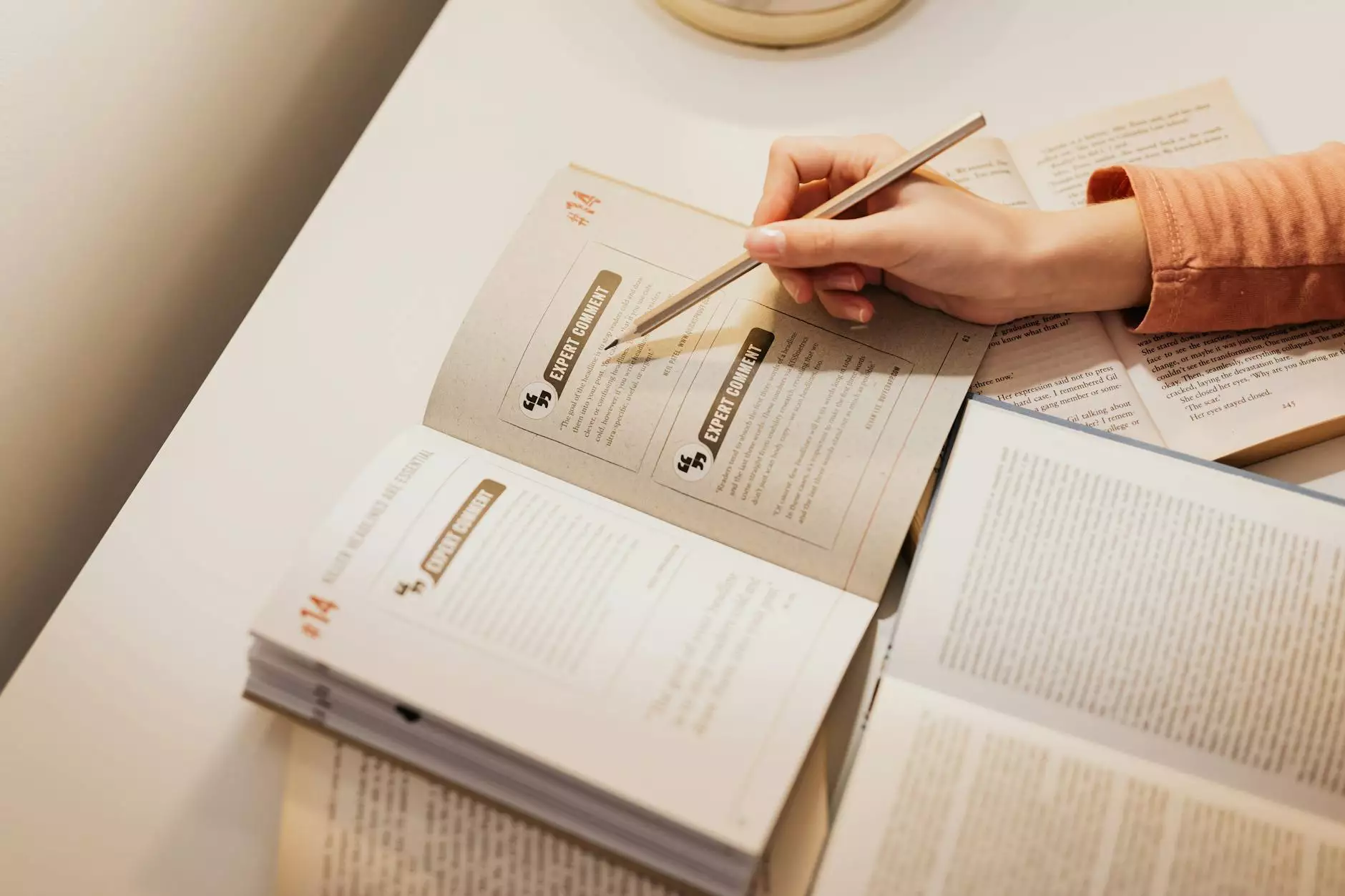
Understanding Data Annotation
Data annotation is a crucial aspect of machine learning and artificial intelligence, involving the labeling of data to train algorithms and create models. It serves as the foundation of various applications in computer vision, natural language processing, and more. As the demand for data-driven insights grows, the need for effective data annotation tools becomes imperative. This is where bounding boxes come into play.
The Role of Bounding Boxes in Data Annotation
Bounding boxes are rectangular borders that can be drawn around objects in images and videos to identify and localize them. They are integral to the process of data annotation, particularly in the realm of computer vision. Here’s why bounding boxes are essential:
- Object Detection: By placing bounding boxes around objects, machine learning models can learn to detect and classify these objects accurately.
- Localization: Bounding boxes provide spatial information about where in an image an object is located, which is crucial for understanding the context.
- Data Quality: High-quality annotations using bounding boxes improve the reliability of the data that's being fed into models, enhancing overall performance.
Types of Bounding Boxes
There are various types of bounding boxes employed in data annotation, each serving different purposes:
1. Tight Bounding Boxes
Tight bounding boxes closely fit around the contours of an object, minimizing the background area. They are particularly useful for tasks that require high precision, such as identifying vehicles in traffic images.
2. Loose Bounding Boxes
Loose bounding boxes encompass a broader area, which may include unwanted background. They are generally easier to create and useful for initial data gathering phases or when rapid annotations are needed.
3. 3D Bounding Boxes
3D bounding boxes extend the concept into three dimensions, useful in applications like autonomous driving where understanding the volume and position of objects relative to the vehicle is essential.
Implementing a Bounding Box Annotation Tool
To leverage the benefits of bounding boxes in your data annotation tasks, implementing a sophisticated data annotation tool is crucial. Here’s how to go about it:
Choosing the Right Platform
Select a data annotation platform that offers intuitive user interfaces and robust features. Platforms like Keylabs.ai provide advanced functionalities for bounding box annotations, making the process efficient and simple.
Streamlined Workflow
Adopt a streamlined workflow that enables your annotators to create bounding boxes quickly. Features such as keyboard shortcuts, automatic detection of objects, and bulk annotation tools can save time and reduce errors.
Collaboration and Review
Facilitate collaboration among team members and establish a review process to maintain the quality of annotations. Peer reviews can help identify inconsistencies and improve the quality of the bounding boxes generated.
How Keylabs.ai Enhances the Annotation Process
Keylabs.ai stands at the forefront of data annotation technology, providing a comprehensive platform that simplifies the creation of bounding boxes:
- User-Friendly Interface: The platform features a user-friendly design that makes it accessible for annotators of all skill levels.
- Automated Detection: Keylabs.ai utilizes advanced algorithms to suggest bounding boxes, reducing the manual workload of annotators.
- Multi-Format Support: The tool supports various formats and integrates seamlessly with existing data pipelines.
Best Practices for Bounding Box Annotation
To ensure quality results from your bounding box annotations, follow these best practices:
1. Define Clear Guidelines
Establish clear annotation guidelines that outline how bounding boxes should be drawn for specific objects. This consistency will improve the overall quality of your dataset.
2. Use High-Quality Images
Ensure that the images used for annotation are of high quality. Poorly lit or blurry images can lead to inaccuracies in placement and sizing of bounding boxes.
3. Regularly Review Annotations
Incorporate a feedback loop where annotations are regularly reviewed and corrected. This practice will help in fine-tuning the skills of annotators and improving dataset integrity.
Challenges in Bounding Box Annotation
While bounding boxes are valuable, challenges can arise during the annotation process. Addressing these challenges is critical for maximizing the effectiveness of your annotations:
1. Object Occlusion
When objects are partially obscured, annotators may struggle to accurately create bounding boxes. Utilizing tools with adjustable box sizes can mitigate this issue by allowing more versatile annotations.
2. Complex Scenes
In scenarios with multiple overlapping objects, determining the right boundaries can be complex. Annotators must be trained to handle such cases, possibly utilizing techniques such as layered annotations.
3. Time Constraints
Annotation can be time-consuming, especially with large datasets. Efficient tools like those offered by Keylabs.ai can significantly reduce the time from hours to minutes, allowing teams to focus on accuracy.
The Future of Bounding Boxes in AI
As artificial intelligence continues to evolve, the role of bounding boxes in data annotation is likely to expand. Advances in machine learning techniques may lead to new methodologies that enhance the accuracy and efficiency of bounding box annotations.
Additionally, the integration of artificial intelligence in annotation tools could eventually lead to fully automated processes where bounding boxes are generated without human intervention, although human oversight will always be necessary to ensure quality.
Conclusion
In conclusion, bounding boxes play an essential role in data annotation, providing a robust solution for identifying and localizing objects in images. By leveraging platforms like Keylabs.ai and following best practices, businesses can enhance their data annotation processes, resulting in higher quality datasets that power effective machine learning models.
The landscape of data annotation is ever-evolving, and with advancements in technology, the future looks promising. Embrace the changes, and maximize the potential of bounding box annotations in your business today.